2022. 1. 5. 10:30ㆍpaper-of-the-day
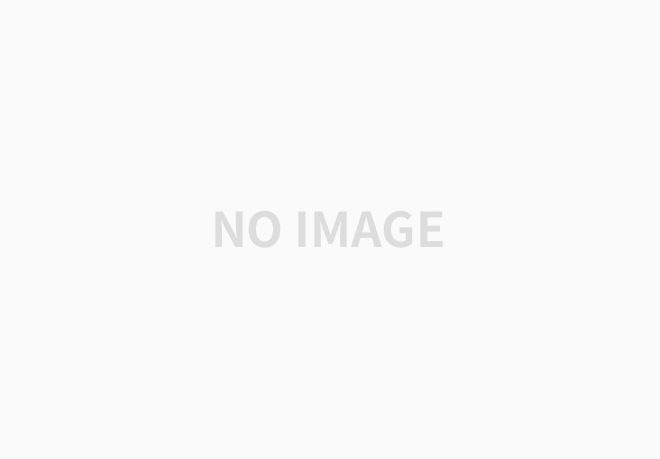
Robust Natural Language Processing: Recent Advances, Challenges, and Future Directions
Recent natural language processing (NLP) techniques have accomplished high performance on benchmark datasets, primarily due to the significant improvement in the performance of deep learning. The advances in the research community have led to great enhancements in state-of-the-art production systems for NLP tasks, such as virtual assistants, speech recognition, and sentiment analysis. However, such NLP systems still often fail when tested with adversarial attacks. The initial lack of robustness exposed troubling gaps in current models' language understanding capabilities, creating problems when NLP systems are deployed in real life. In this paper, we present a structured overview of NLP robustness research by summarizing the literature in a systemic way across various dimensions. We then take a deep-dive into the various dimensions of robustness, across techniques, metrics, embeddings, and benchmarks. Finally, we argue that robustness should be multi-dimensional, provide insights into current research, identify gaps in the literature to suggest directions worth pursuing to address these gaps.
최근 NLP(Natural Language Processing) 기술은 높은 성과를 거두었다. 벤치마크 데이터셋의 성능 향상으로 인한 성능 향상 깊은 학습의 수행에서. 연구 커뮤니티의 발전 NLP를 위한 최첨단 생산 시스템을 크게 개선했다. 가상 비서, 음성 인식 및 정서 분석과 같은 작업. 그러나 그러한 NLP 시스템은 적대적인 방법으로 테스트할 때 여전히 실패하는 경우가 많다. 공격. 초기의 견고성 부족은 전류의 골치 아픈 틈을 노출시켰다. 모델의 언어 이해 능력, NLP 시스템 문제 발생 실생활에서 전개됩니다. 본 논문에서, 우리는 다음에 대한 구조화된 개요를 제시한다. 전체적으로 체계적인 방법으로 문헌을 요약한 NLP 건전성 연구 다양한 차원 그리고 나서 우리는 다양한 차원으로 깊게 잠수한다. 기술, 메트릭, 임베딩 및 벤치마크 전반에 걸친 견고성. 드디어 저희가. 강건성이 다차원적이어야 한다고 주장하고, 에 대한 통찰력을 제공한다 현재 연구, 가치 있는 방향을 제시하기 위해 문헌의 차이를 확인한다 이러한 격차를 해소하기 위해 노력하고 있습니다.
Topical Classification of Food Safety Publications with a Knowledge Base
The vast body of scientific publications presents an increasing challenge of finding those that are relevant to a given research question, and making informed decisions on their basis. This becomes extremely difficult without the use of automated tools. Here, one possible area for improvement is automatic classification of publication abstracts according to their topic. This work introduces a novel, knowledge base-oriented publication classifier. The proposed method focuses on achieving scalability and easy adaptability to other domains. Classification speed and accuracy are shown to be satisfactory, in the very demanding field of food safety. Further development and evaluation of the method is needed, as the proposed approach shows much potential.
과학 출판물의 방대한 본체는 점점 더 많은 도전을 제시한다. 주어진 연구 문제와 관련된 것들을 찾고, 만든다. 정보에 입각한 결정을 내릴 수 있습니다. 이 작업은 다음과 같은 기능 없이는 매우 어려워집니다. 자동화된 도구 사용. 여기서 개선할 수 있는 한 가지 영역은 자동입니다. 출판물의 분류는 주제에 따라 추상화된다. 이 작품 새로운 기술 자료 지향 출판 분류기를 소개합니다. 그 제안된 방법은 다른 방법에 대한 확장성 및 손쉬운 적응성을 달성하는 데 초점을 맞춘다. 도메인. 분류 속도와 정확도가 만족스러운 것으로 나타났다. 매우 까다로운 식품 안전 분야 추가 개발 및 평가 제안된 접근방식이 많은 가능성을 보여주기 때문에 방법이 필요하다.
Robust Natural Language Processing: Recent Advances, Challenges, and Future Directions
Recent natural language processing (NLP) techniques have accomplished high performance on benchmark datasets, primarily due to the significant improvement in the performance of deep learning. The advances in the research community have led to great enhancements in state-of-the-art production systems for NLP tasks, such as virtual assistants, speech recognition, and sentiment analysis. However, such NLP systems still often fail when tested with adversarial attacks. The initial lack of robustness exposed troubling gaps in current models' language understanding capabilities, creating problems when NLP systems are deployed in real life. In this paper, we present a structured overview of NLP robustness research by summarizing the literature in a systemic way across various dimensions. We then take a deep-dive into the various dimensions of robustness, across techniques, metrics, embeddings, and benchmarks. Finally, we argue that robustness should be multi-dimensional, provide insights into current research, identify gaps in the literature to suggest directions worth pursuing to address these gaps.
최근 NLP(Natural Language Processing) 기술은 높은 성과를 거두었다. 벤치마크 데이터셋의 성능 향상으로 인한 성능 향상 깊은 학습의 수행에서. 연구 커뮤니티의 발전 NLP를 위한 최첨단 생산 시스템을 크게 개선했다. 가상 비서, 음성 인식 및 정서 분석과 같은 작업. 그러나 그러한 NLP 시스템은 적대적인 방법으로 테스트할 때 여전히 실패하는 경우가 많다. 공격. 초기의 견고성 부족은 전류의 골치 아픈 틈을 노출시켰다. 모델의 언어 이해 능력, NLP 시스템 문제 발생 실생활에서 전개됩니다. 본 논문에서, 우리는 다음에 대한 구조화된 개요를 제시한다. 전체적으로 체계적인 방법으로 문헌을 요약한 NLP 건전성 연구 다양한 차원 그리고 나서 우리는 다양한 차원으로 깊게 잠수한다. 기술, 메트릭, 임베딩 및 벤치마크 전반에 걸친 견고성. 드디어 저희가. 강건성이 다차원적이어야 한다고 주장하고, 에 대한 통찰력을 제공한다 현재 연구, 가치 있는 방향을 제시하기 위해 문헌의 차이를 확인한다 이러한 격차를 해소하기 위해 노력하고 있습니다.
'paper-of-the-day' 카테고리의 다른 글
[2022-01-07] 오늘의 자연어처리 (0) | 2022.01.07 |
---|---|
[2022-01-06] 오늘의 자연어처리 (0) | 2022.01.06 |
[2022-01-04] 오늘의 자연어처리 (0) | 2022.01.04 |
[2022-01-02] 오늘의 자연어처리 (0) | 2022.01.02 |
[2021-12-30] 오늘의 자연어처리 (0) | 2021.12.30 |