2022. 1. 13. 10:30ㆍpaper-of-the-day
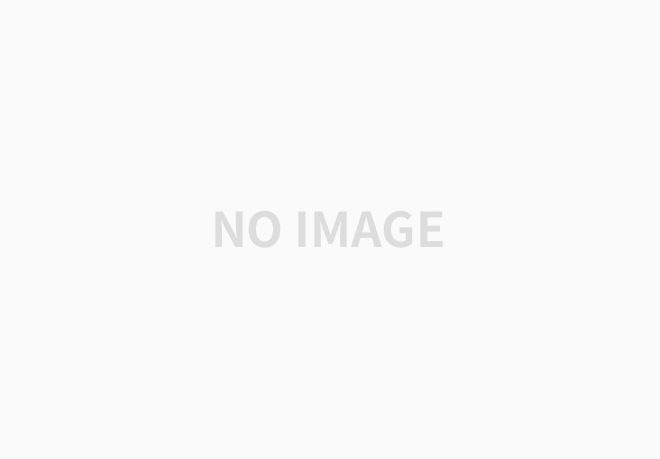
Multimodal Representations Learning Based on Mutual Information Maximization and Minimization and Identity Embedding for Multimodal Sentiment Analysis
Multimodal sentiment analysis (MSA) is a fundamental complex research problem due to the heterogeneity gap between different modalities and the ambiguity of human emotional expression. Although there have been many successful attempts to construct multimodal representations for MSA, there are still two challenges to be addressed: 1) A more robust multimodal representation needs to be constructed to bridge the heterogeneity gap and cope with the complex multimodal interactions, and 2) the contextual dynamics must be modeled effectively throughout the information flow. In this work, we propose a multimodal representation model based on Mutual information Maximization and Minimization and Identity Embedding (MMMIE). We combine mutual information maximization between modal pairs, and mutual information minimization between input data and corresponding features to mine the modal-invariant and task-related information. Furthermore, Identity Embedding is proposed to prompt the downstream network to perceive the contextual information. Experimental results on two public datasets demonstrate the effectiveness of the proposed model.
MSA(Multimodal 정서 분석)는 근본적인 복잡한 연구 문제이다. 다른 양식과 의 모호성 사이의 이질성 격차 때문에 인간의 감정 표현 비록 많은 성공적인 시도가 있었지만 MSA에 대한 다중 모드 표현을 구성하기 위해, 여전히 두 가지 과제가 있다. 다루어야 할 사항: 1) 보다 강력한 멀티모달 표현은 이질성 격차를 해소하고 복합체에 대처하기 위해 구성되었다. 다중 모드 상호 작용, 2) 상황 역학을 모델링해야 한다. 정보 흐름 전반에 걸쳐 효과적으로 활용합니다. 본 연구에서는, 우리는 다음과 같은 것을 제안한다. 상호 정보 극대화에 기초한 다중 모드 표현 모델 및 최소화 및 정체성 임베딩(MMIE)입니다. 우리는 상호 정보를 결합한다. 모달 쌍 간의 최대화, 그리고 사이의 상호 정보 최소화 입력 데이터 및 해당 기능을 마이닝하여 모달-중요 및 작업 관련 정보. 게다가, 아이덴티티 임베딩은 다음을 촉진하기 위해 제안되었다. 상황에 맞는 정보를 인식하기 위한 다운스트림 네트워크. 실험 두 개의 공개 데이터셋에 대한 결과는 제안된 데이터셋의 효과를 입증한다. 본보기로 하다
BERT for Sentiment Analysis: Pre-trained and Fine-Tuned Alternatives
BERT has revolutionized the NLP field by enabling transfer learning with large language models that can capture complex textual patterns, reaching the state-of-the-art for an expressive number of NLP applications. For text classification tasks, BERT has already been extensively explored. However, aspects like how to better cope with the different embeddings provided by the BERT output layer and the usage of language-specific instead of multilingual models are not well studied in the literature, especially for the Brazilian Portuguese language. The purpose of this article is to conduct an extensive experimental study regarding different strategies for aggregating the features produced in the BERT output layer, with a focus on the sentiment analysis task. The experiments include BERT models trained with Brazilian Portuguese corpora and the multilingual version, contemplating multiple aggregation strategies and open-source datasets with predefined training, validation, and test partitions to facilitate the reproducibility of the results. BERT achieved the highest ROC-AUC values for the majority of cases as compared to TF-IDF. Nonetheless, TF-IDF represents a good trade-off between the predictive performance and computational cost.
BERT는 NLP 분야를 혁신적으로 변화시켰다. 복잡한 텍스트 패턴을 캡처할 수 있는 대규모 언어 모델, 도달 표현 가능한 수의 NLP 애플리케이션을 위한 최신 기술. 텍스트의 경우 분류 작업, BERT는 이미 광범위하게 탐색되었다. 하지만 에 의해 제공되는 다양한 임베딩에 더 잘 대처하는 방법과 같은 측면 BERT 출력 계층 및 다국어 대신 언어별 사용 모델들은 문헌에서 잘 연구되지 않는다, 특히 브라질 사람들에게 포르투갈어. 이 글의 목적은 광범위한 작업을 수행하는 것이다. 특징을 종합하기 위한 다양한 전략에 관한 실험적 연구 감정 분석 작업에 중점을 두고 BERT 출력 계층에서 생성된다. 실험은 브라질 포르투갈 말뭉치로 훈련된 BERT 모델을 포함한다. 다국어 버전, 다중 집계 전략 및 사전 정의된 교육, 검증 및 테스트 파티션이 있는 오픈 소스 데이터셋 결과의 재현성을 용이하게 하기 위해. BERT는 최고를 달성했다. TF-IDF와 비교하여 대부분의 경우 ROC-AUC 값. 그럼에도 불구하고. TF-IDF는 예측 성과와 예측 성과 사이의 좋은 균형을 나타낸다. 계산 비용
Prior Knowledge Enhances Radiology Report Generation
Radiology report generation aims to produce computer-aided diagnoses to alleviate the workload of radiologists and has drawn increasing attention recently. However, previous deep learning methods tend to neglect the mutual influences between medical findings, which can be the bottleneck that limits the quality of generated reports. In this work, we propose to mine and represent the associations among medical findings in an informative knowledge graph and incorporate this prior knowledge with radiology report generation to help improve the quality of generated reports. Experiment results demonstrate the superior performance of our proposed method on the IU X-ray dataset with a ROUGE-L of 0.384$\pm$0.007 and CIDEr of 0.340$\pm$0.011. Compared with previous works, our model achieves an average of 1.6% improvement (2.0% and 1.5% improvements in CIDEr and ROUGE-L, respectively). The experiments suggest that prior knowledge can bring performance gains to accurate radiology report generation. We will make the code publicly available at this https URL.
방사선과 보고서 생성은 다음 항목에 대한 컴퓨터 지원 진단의 생성을 목표로 한다. 방사선 전문의의 업무량을 줄이고 점점 더 많은 관심을 끌고 있다. 최근에 그러나 이전의 딥러닝 방법은 상호관계를 무시하는 경향이 있다. 제한되는 병목 현상이 될 수 있는 의료 발견 사이의 영향 생성된 보고서의 품질. 이 작품에서, 우리는 광산을 제안하고 유익한 지식으로 의학 발견 사이의 연관성을 나타낸다. 그래프로 표시하고 이 사전 지식을 방사선과 보고서 생성과 통합한다. 생성된 보고서의 품질을 향상시키는 데 도움이 됩니다. 실험 결과는 다음과 같이 입증한다. IU X선 데이터 세트에 대해 제안된 방법의 우수한 성능 ROUGE-L은 0.384$\pm$0.007이고 CIDEr은 0.340$\pm$0.011입니다. 이전과 비교 작동합니다. 모델이 평균 1.6% 개선(2.0% 및 1.5%). 각각 CIDEr 및 ROUGE-L의 개선). 실험 결과 다음과 같다. 사전 지식이 정확한 방사선 보고서에 성능 향상을 가져올 수 있다. 시대 다음에서 코드를 공개적으로 사용할 수 있도록 할 것입니다. 이 https URL.
'paper-of-the-day' 카테고리의 다른 글
[2022-01-17] 오늘의 자연어처리 (0) | 2022.01.17 |
---|---|
[2022-01-14] 오늘의 자연어처리 (0) | 2022.01.14 |
[2022-01-12] 오늘의 자연어처리 (0) | 2022.01.12 |
[2022-01-11] 오늘의 자연어처리 (0) | 2022.01.11 |
[2022-01-10] 오늘의 자연어처리 (0) | 2022.01.10 |