2022. 2. 10. 10:30ㆍpaper-of-the-day
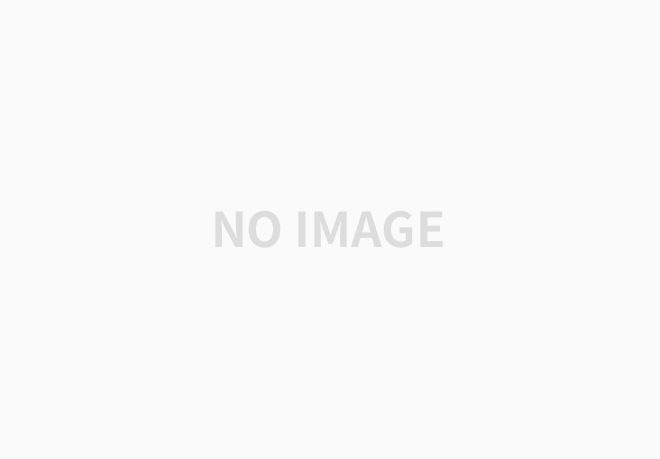
Selecting Seed Words for Wordle using Character Statistics
Wordle, a word guessing game rose to global popularity in the January of 2022. The goal of the game is to guess a five-letter English word within six tries. Each try provides the player with hints by means of colour changing tiles which inform whether or not a given character is part of the solution as well as, in cases where it is part of the solution, whether or not it is in the correct placement. Numerous attempts have been made to find the best starting word and best strategy to solve the daily wordle. This study uses character statistics of five-letter words to determine the best three starting words.
워들, 단어 추측 게임은 1월에 세계적인 인기를 얻었다. 이 게임의 목표는 여섯 글자 안에 다섯 글자의 영어 단어를 맞추는 것이다. 시도한다. 각각의 시도는 플레이어에게 색상 변화를 통해 힌트를 제공한다. 주어진 문자가 솔루션의 일부인지 여부를 알려주는 타일: 그리고, 그것이 해결책의 일부인 경우, 그것이 그 안에 있든 아니든, 정확한 배치 최고의 출발을 찾기 위한 수많은 시도가 있었다. 일상적인 문제를 해결하기 위한 단어와 최선의 전략. 이 연구는 문자를 사용합니다. 다섯 글자로 된 단어의 통계를 사용하여 가장 좋은 세 개의 시작 단어를 결정합니다.
Self-Supervised Representation Learning for Speech Using Visual Grounding and Masked Language Modeling
In this paper, we describe our submissions to the ZeroSpeech 2021 Challenge and SUPERB benchmark. Our submissions are based on the recently proposed FaST-VGS model, which is a Transformer-based model that learns to associate raw speech waveforms with semantically related images, all without the use of any transcriptions of the speech. Additionally, we introduce a novel extension of this model, FaST-VGS+, which is learned in a multi-task fashion with a masked language modeling objective in addition to the visual grounding objective. On ZeroSpeech 2021, we show that our models perform competitively on the ABX task, outperform all other concurrent submissions on the Syntactic and Semantic tasks, and nearly match the best system on the Lexical task. On the SUPERB benchmark, we show that our models also achieve strong performance, in some cases even outperforming the popular wav2vec2.0 model.
본 논문에서, 우리는 ZeroSpeech 2021 챌린지에 제출한 내용을 설명한다. 및 SUPERECT 벤치마크. 우리의 제출은 최근에 제안된 것에 기초한다. FaST-VGS 모델은 원시 연관성을 학습하는 트랜스포머 기반 모델입니다. 의미론적으로 관련된 영상이 있는 음성 파형, 아무 것도 사용하지 않고 연설문 사본 추가적으로, 우리는 의 새로운 확장을 소개한다. 이 모델, FaST-VGS+는 마스크가 있는 다중 작업 방식으로 학습된다. 언어 모델링 목표 및 시각적 접지 목표. 켜짐 ZeroSpeech 2021은 ABX 작업에서 우리의 모델이 경쟁적으로 수행된다는 것을 보여준다. 통사론 및 시맨틱에 대한 다른 모든 동시 제출을 능가한다. 작업 및 어휘 작업에 대한 최상의 시스템과 거의 일치합니다. 최고를 타고 벤치마크, 우리는 우리의 모델들이 또한 어떤 면에서 강력한 성능을 달성한다는 것을 보여준다. 심지어 인기 있는 wav2vec2.0 모델을 능가하는 케이스도 있다.
Do Language Models Learn Position-Role Mappings?
How is knowledge of position-role mappings in natural language learned? We explore this question in a computational setting, testing whether a variety of well-performing pertained language models (BERT, RoBERTa, and DistilBERT) exhibit knowledge of these mappings, and whether this knowledge persists across alternations in syntactic, structural, and lexical alternations. In Experiment 1, we show that these neural models do indeed recognize distinctions between theme and recipient roles in ditransitive constructions, and that these distinct patterns are shared across construction type. We strengthen this finding in Experiment 2 by showing that fine-tuning these language models on novel theme- and recipient-like tokens in one paradigm allows the models to make correct predictions about their placement in other paradigms, suggesting that the knowledge of these mappings is shared rather than independently learned. We do, however, observe some limitations of this generalization when tasks involve constructions with novel ditransitive verbs, hinting at a degree of lexical specificity which underlies model performance.
자연어에서의 위치 역할 매핑에 대한 지식은 어떻게 학습되는가? 우리가 다양한 문제를 테스트하면서 계산 환경에서 이 문제를 탐색합니다. 성능이 우수한 지속 언어 모델(BERT, RoBERTa 및 DistilBERT) 이러한 매핑에 대한 지식과 이 지식이 전체에 걸쳐 지속되는지 여부를 보여줍니다. 통사적, 구조적, 어휘적 교류의 변화. 실험 중 1, 우리는 이 신경 모델들이 정말로 사이의 차이를 인식한다는 것을 보여준다. 주제 및 수신자 역할, 그리고 이러한 것들이 구별되는 패턴은 시공 유형 전반에 걸쳐 공유됩니다. 우리는 이것을 강화한다. 실험 2에서 이러한 언어 모델을 미세 조정하는 것을 보여줌으로써 발견 하나의 패러다임에서 새로운 테마와 수신자 같은 토큰은 모델들이 다음을 가능하게 한다. 다른 패러다임에서의 그들의 배치에 대해 올바른 예측을 하고, 시사한다. 이러한 매핑에 대한 지식이 독립적으로 공유되는 것이 아니라 공유된다는 것 배웠다 그러나 우리는 다음과 같은 경우 이 일반화의 몇 가지 한계를 관찰한다. 과제는 정도를 암시하는 새로운 이타동사 구성을 포함한다. 모델 성능의 기초가 되는 어휘 특이성의.
'paper-of-the-day' 카테고리의 다른 글
[2022-02-14] 오늘의 자연어처리 (0) | 2022.02.14 |
---|---|
[2022-02-11] 오늘의 자연어처리 (0) | 2022.02.11 |
[2022-02-09] 오늘의 자연어처리 (0) | 2022.02.09 |
[2022-02-08] 오늘의 자연어처리 (0) | 2022.02.08 |
[2022-02-07] 오늘의 자연어처리 (0) | 2022.02.07 |