2022. 3. 15. 10:30ㆍpaper-of-the-day
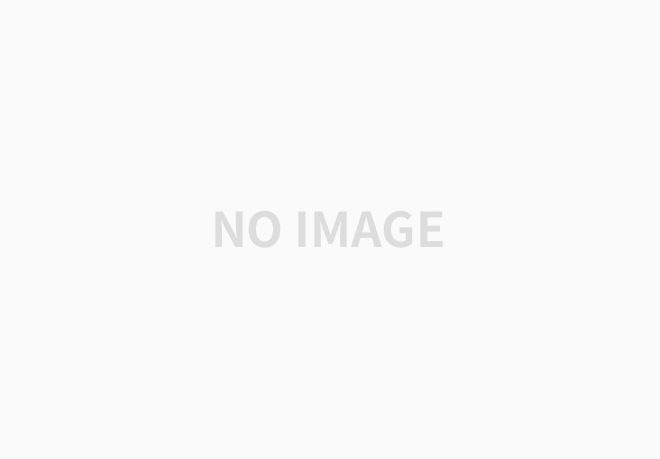
IndicNLG Suite: Multilingual Datasets for Diverse NLG Tasks in Indic Languages
In this paper, we present the IndicNLG suite, a collection of datasets for benchmarking Natural Language Generation (NLG) for 11 Indic languages. We focus on five diverse tasks, namely, biography generation using Wikipedia infoboxes (WikiBio), news headline generation, sentence summarization, question generation and paraphrase generation. We describe the process of creating the datasets and present statistics of the dataset, following which we train and report a variety of strong monolingual and multilingual baselines that leverage pre-trained sequence-to-sequence models and analyze the results to understand the challenges involved in Indic language NLG. To the best of our knowledge, this is the first NLG dataset for Indic languages and also the largest multilingual NLG dataset. Our methods can also be easily applied to modest-resource languages with reasonable monolingual and parallel corpora, as well as corpora containing structured data like Wikipedia. We hope this dataset spurs research in NLG on diverse languages and tasks, particularly for Indic languages. The datasets and models are publicly available at this https URL.
본 논문에서, 우리는 다음을 위한 데이터셋 모음인 IndicNLG 제품군을 제시한다. 11개 지표 언어에 대한 NLG(Natural Language Generation) 벤치마킹. 우리는 집중한다 다섯 가지 다양한 과제, 즉 위키백과 정보 상자를 이용한 전기 생성에 대해 (WikiBio), 뉴스 헤드라인 생성, 문장 요약, 질문 생성 및 번역 생성. 우리는 생성 과정을 설명한다. 데이터셋과 데이터셋의 통계를 제시합니다, 그리고 우리가 교육하고, 다음을 활용하는 다양한 강력한 단일 언어 및 다국어 기준선을 보고한다 사전 교육을 받은 시퀀스-투-시퀀스 모델 및 결과를 분석하여 이해 인도어 NLG에 관련된 과제들 우리가 아는 한, 이것은 Indic 언어를 위한 최초의 NLG 데이터 세트이며 또한 가장 큰 데이터 세트입니다. 다국어 NLG 데이터 세트. 우리의 방법은 또한 쉽게 적용될 수 있다. 로서 합당한 단일어 및 병렬 코퍼스를 가진 중간 자원 언어들 위키피디아와 같은 구조화된 데이터를 포함하고 있는 말뭉치도요 이 데이터 집합이 필요합니다. 특히 Indic을 위한 다양한 언어와 작업에 대한 NLG 연구를 촉진한다. 언어들 데이터셋과 모델은 다음에서 공개적으로 제공된다. 이 https URL.
Parameter-Free Attentive Scoring for Speaker Verification
This paper presents a novel study of parameter-free attentive scoring for speaker verification. Parameter-free scoring provides the flexibility of comparing speaker representations without the need of an accompanying parametric scoring model. Inspired by the attention component in Transformer neural networks, we propose a variant of the scaled dot product attention mechanism to compare enrollment and test segment representations. In addition, this work explores the effect on performance of (i) different types of normalization, (ii) independent versus tied query/key estimation, (iii) varying the number of key-value pairs and (iv) pooling multiple enrollment utterance statistics. Experimental results for a 4 task average show that a simple parameter-free attentive scoring mechanism can improve the average EER by 10% over the best cosine similarity baseline.
본 논문은 다음에 대한 매개 변수 없는 주의 깊은 채점에 대한 새로운 연구를 제시한다. 화자 검증 매개 변수가 없는 스코어링은 다음과 같은 유연성을 제공합니다. 동반할 필요 없이 화자의 표현 비교 파라메트릭 스코어링 모델. 트랜스포머의 어텐션 컴포넌트에서 영감을 받았습니다. 신경망, 우리는 스케일링된 도트 제품 주의의 변형을 제안한다. 등록 및 테스트 세그먼트 표현을 비교하는 메커니즘. 게다가. 이 연구는 (i) 다른 유형의 의 성능에 미치는 영향을 탐구한다 정규화, (ii) 독립 대 동점 쿼리/키 추정, (iii) 변동 키-값 쌍의 수 및 (iv) 다중 등록 발화 풀링 통계학 4개의 작업 평균에 대한 실험 결과는 간단한 것을 보여준다. 매개 변수가 없는 주의 깊은 채점 메커니즘은 평균 EER을 10%까지 향상시킬 수 있다. 코사인 유사도 기준선을 초과한다.
Contextualized Sensorimotor Norms: multi-dimensional measures of sensorimotor strength for ambiguous English words, in context
Most large language models are trained on linguistic input alone, yet humans appear to ground their understanding of words in sensorimotor experience. A natural solution is to augment LM representations with human judgments of a word's sensorimotor associations (e.g., the Lancaster Sensorimotor Norms), but this raises another challenge: most words are ambiguous, and judgments of words in isolation fail to account for this multiplicity of meaning (e.g., "wooden table" vs. "data table"). We attempted to address this problem by building a new lexical resource of contextualized sensorimotor judgments for 112 English words, each rated in four different contexts (448 sentences total). We show that these ratings encode overlapping but distinct information from the Lancaster Sensorimotor Norms, and that they also predict other measures of interest (e.g., relatedness), above and beyond measures derived from BERT. Beyond shedding light on theoretical questions, we suggest that these ratings could be of use as a "challenge set" for researchers building grounded language models.
대부분의 큰 언어 모델들은 언어 입력만으로 훈련되지만, 여전히 인간들은 감각 운동 경험에서 단어의 이해를 뒷받침하는 것으로 보인다. a 자연스러운 해결책은 인간의 판단으로 LM 표현을 증가시키는 것이다 워드의 센서이모터 연관성(예: Lancaster Sensorimotor Normal), 이것은 또 다른 도전을 제기한다: 대부분의 단어들은 모호하고, 단어들에 대한 판단 단독으로 이 의미의 다양성을 설명하지 못한다(예: "discluse. 테이블" 대 데이터 테이블). 우리는 이 문제를 해결하기 위해 a를 구축했습니다. 112개의 영어에 대한 문맥화된 센서이모터 판단의 새로운 어휘 자원 단어, 각각 4개의 다른 맥락에서 평가됩니다(총 448문장). 우리는 보여준다 이 등급들이 겹치지만 구별되는 정보를 암호화하는 것 Lancaster Sensorimotor 규범, 그리고 그들은 또한 다른 측정들을 예측합니다. 관심(예: 관련성), BERT에서 도출된 측정 초과. 이론적 질문을 조명하는 것 이상으로, 우리는 이러한 등급을 제안한다. 기초 언어를 만드는 연구자들을 위한 "모서리 집합"으로 사용될 수 있다. 모델들.
'paper-of-the-day' 카테고리의 다른 글
[2022-03-21] 오늘의 자연어처리 (0) | 2022.03.21 |
---|---|
[2022-03-16] 오늘의 자연어처리 (0) | 2022.03.16 |
[2022-03-14] 오늘의 자연어처리 (0) | 2022.03.14 |
[2022-03-07] 오늘의 자연어처리 (0) | 2022.03.07 |
[2022-03-04] 오늘의 자연어처리 (0) | 2022.03.04 |