2022. 1. 26. 10:30ㆍpaper-of-the-day
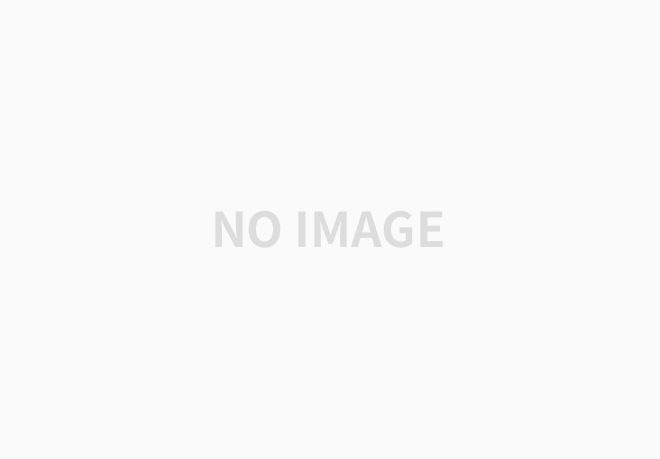
Bias in Automated Speaker Recognition
Automated speaker recognition uses data processing to identify speakers by their voice. Today, automated speaker recognition technologies are deployed on billions of smart devices and in services such as call centres. Despite their wide-scale deployment and known sources of bias in face recognition and natural language processing, bias in automated speaker recognition has not been studied systematically. We present an in-depth empirical and analytical study of bias in the machine learning development workflow of speaker verification, a voice biometric and core task in automated speaker recognition. Drawing on an established framework for understanding sources of harm in machine learning, we show that bias exists at every development stage in the well-known VoxCeleb Speaker Recognition Challenge, including model building, implementation, and data generation. Most affected are female speakers and non-US nationalities, who experience significant performance degradation. Leveraging the insights from our findings, we make practical recommendations for mitigating bias in automated speaker recognition, and outline future research directions.
자동 화자 인식은 데이터 처리를 사용하여 화자를 식별한다. 그들의 목소리. 오늘날, 자동화된 화자 인식 기술은 다음과 같이 배치된다. 수십억 개의 스마트 기기 및 콜 센터와 같은 서비스에 사용됩니다. 그럼에도 불구하고 얼굴 인식 및 자연적 편견의 광범위한 배치 및 알려진 원인 언어 처리, 자동 화자 인식의 편향은 연구되지 않았다. 질서정연하게 우리는 편향에 대한 심층적인 경험적, 분석적 연구를 제시한다. 스피커 검증의 기계 학습 개발 워크플로우에서, 음성 자동 스피커 인식의 생체 인식 및 핵심 작업. 그림 그리기 기계 학습에서 위해의 원천을 이해하기 위한 확립된 프레임워크, 우리는 잘 알려진 VoxCeleb의 모든 개발 단계에서 편향이 존재한다는 것을 보여준다. 모델 구축, 구현, 그리고 화자 인식 데이터 생성 대부분의 피해자들은 여성 화자들과 미국 국적이 아닌 사람들입니다 심각한 성능 저하를 경험합니다. 통찰력 활용 우리의 발견으로부터, 우리는 편향을 완화하기 위한 실질적인 권고안을 만든다 자동 화자 인식 및 향후 연구 방향의 개요를 제공합니다.
Description-Driven Task-Oriented Dialog Modeling
Task-oriented dialogue (TOD) systems are required to identify key information from conversations for the completion of given tasks. Such information is conventionally specified in terms of intents and slots contained in task-specific ontology or schemata. Since these schemata are designed by system developers, the naming convention for slots and intents is not uniform across tasks, and may not convey their semantics effectively. This can lead to models memorizing arbitrary patterns in data, resulting in suboptimal performance and generalization. In this paper, we propose that schemata should be modified by replacing names or notations entirely with natural language descriptions. We show that a language description-driven system exhibits better understanding of task specifications, higher performance on state tracking, improved data efficiency, and effective zero-shot transfer to unseen tasks. Following this paradigm, we present a simple yet effective Description-Driven Dialog State Tracking (D3ST) model, which relies purely on schema descriptions and an "index-picking" mechanism. We demonstrate the superiority in quality, data efficiency and robustness of our approach as measured on the MultiWOZ (Budzianowski et al.,2018), SGD (Rastogi et al., 2020), and the recent SGD-X (Lee et al., 2021) benchmarks.
주요 정보를 식별하기 위해 작업 지향 대화(TOD) 시스템이 필요하다. 주어진 임무를 완수하기 위한 대화로부터. 그러한 정보는 일반적으로 에 포함된 의도 및 슬롯 측면에서 명시되어 있다. 태스크별 온톨로지 또는 스키마. 이러한 스키마는 시스템에 의해 설계되기 때문에 developers, 슬롯 및 의도에 대한 명명 규칙이 전체 간에 균일하지 않습니다. 작업 및 그 의미를 효과적으로 전달하지 못할 수 있다. 이로 인해 모델이 생성될 수 있습니다. 데이터의 임의 패턴을 암기하여 성능 저하 및 일반화 본 논문에서, 우리는 스키마가 다음과 같이 수정되어야 한다고 제안한다. 이름이나 표기를 완전히 자연어 설명으로 대체한다. 우리가 언어 기술 중심 시스템이 에 대한 더 나은 이해를 나타낸다는 것을 보여준다 태스크 사양, 상태 추적 성능 향상, 데이터 개선 효율성 및 보이지 않는 작업에 대한 효과적인 제로샷 전송. 다음과 같이 패러다임은 간단하지만 효과적인 Description-Driven 대화 상자 상태를 제시한다. 추적(D3)ST) 모델, 스키마 설명 및 기타 정보에 전적으로 의존합니다. "인덱스-레코드" 품질, 데이터의 우수성을 입증합니다. Multi에서 측정된 접근 방식의 효율성과 견고성WOZ (Budzianowski 등, 2018), SGD(Rastogi 등, 2020) 및 최신 SGD-X (Lee 등, 2021) 벤치마크.
Description-Driven Task-Oriented Dialog Modeling
Task-oriented dialogue (TOD) systems are required to identify key information from conversations for the completion of given tasks. Such information is conventionally specified in terms of intents and slots contained in task-specific ontology or schemata. Since these schemata are designed by system developers, the naming convention for slots and intents is not uniform across tasks, and may not convey their semantics effectively. This can lead to models memorizing arbitrary patterns in data, resulting in suboptimal performance and generalization. In this paper, we propose that schemata should be modified by replacing names or notations entirely with natural language descriptions. We show that a language description-driven system exhibits better understanding of task specifications, higher performance on state tracking, improved data efficiency, and effective zero-shot transfer to unseen tasks. Following this paradigm, we present a simple yet effective Description-Driven Dialog State Tracking (D3ST) model, which relies purely on schema descriptions and an "index-picking" mechanism. We demonstrate the superiority in quality, data efficiency and robustness of our approach as measured on the MultiWOZ (Budzianowski et al.,2018), SGD (Rastogi et al., 2020), and the recent SGD-X (Lee et al., 2021) benchmarks.
주요 정보를 식별하기 위해 작업 지향 대화(TOD) 시스템이 필요하다. 주어진 임무를 완수하기 위한 대화로부터. 그러한 정보는 일반적으로 에 포함된 의도 및 슬롯 측면에서 명시되어 있다. 태스크별 온톨로지 또는 스키마. 이러한 스키마는 시스템에 의해 설계되기 때문에 developers, 슬롯 및 의도에 대한 명명 규칙이 전체 간에 균일하지 않습니다. 작업 및 그 의미를 효과적으로 전달하지 못할 수 있다. 이로 인해 모델이 생성될 수 있습니다. 데이터의 임의 패턴을 암기하여 성능 저하 및 일반화 본 논문에서, 우리는 스키마가 다음과 같이 수정되어야 한다고 제안한다. 이름이나 표기를 완전히 자연어 설명으로 대체한다. 우리가 언어 기술 중심 시스템이 에 대한 더 나은 이해를 나타낸다는 것을 보여준다 태스크 사양, 상태 추적 성능 향상, 데이터 개선 효율성 및 보이지 않는 작업에 대한 효과적인 제로샷 전송. 다음과 같이 패러다임은 간단하지만 효과적인 Description-Driven 대화 상자 상태를 제시한다. 추적(D3)ST) 모델, 스키마 설명 및 기타 정보에 전적으로 의존합니다. "인덱스-레코드" 품질, 데이터의 우수성을 입증합니다. Multi에서 측정된 접근 방식의 효율성과 견고성WOZ (Budzianowski 등, 2018), SGD(Rastogi 등, 2020) 및 최신 SGD-X (Lee 등, 2021) 벤치마크.
'paper-of-the-day' 카테고리의 다른 글
[2022-01-28] 오늘의 자연어처리 (0) | 2022.01.28 |
---|---|
[2022-01-27] 오늘의 자연어처리 (0) | 2022.01.27 |
[2022-01-25] 오늘의 자연어처리 (0) | 2022.01.25 |
[2022-01-24] 오늘의 자연어처리 (0) | 2022.01.24 |
[2022-01-21] 오늘의 자연어처리 (0) | 2022.01.21 |