2022. 1. 28. 10:30ㆍpaper-of-the-day
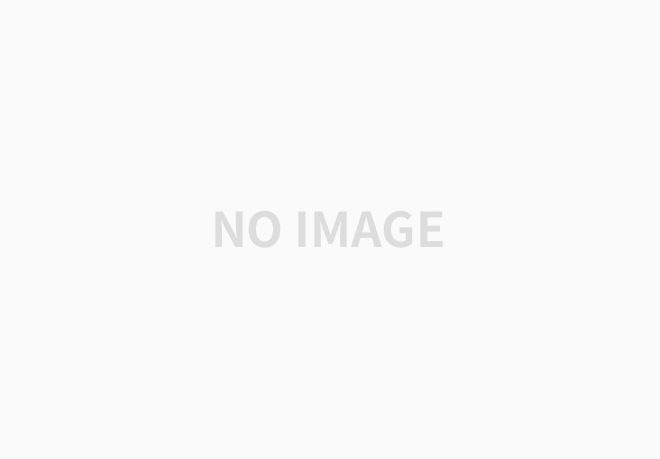
Transfer Learning Approaches for Building Cross-Language Dense Retrieval Models
The advent of transformer-based models such as BERT has led to the rise of neural ranking models. These models have improved the effectiveness of retrieval systems well beyond that of lexical term matching models such as BM25. While monolingual retrieval tasks have benefited from large-scale training collections such as MS MARCO and advances in neural architectures, cross-language retrieval tasks have fallen behind these advancements. This paper introduces ColBERT-X, a generalization of the ColBERT multi-representation dense retrieval model that uses the XLM-RoBERTa (XLM-R) encoder to support cross-language information retrieval (CLIR). ColBERT-X can be trained in two ways. In zero-shot training, the system is trained on the English MS MARCO collection, relying on the XLM-R encoder for cross-language mappings. In translate-train, the system is trained on the MS MARCO English queries coupled with machine translations of the associated MS MARCO passages. Results on ad hoc document ranking tasks in several languages demonstrate substantial and statistically significant improvements of these trained dense retrieval models over traditional lexical CLIR baselines.
BERT와 같은 변압기 기반 모델의 출현은 다음의 성장을 이끌었다. 신경 순위 모델. 이러한 모델은 다음과 같은 효과를 개선했습니다. 다음과 같은 어휘적 용어 매칭 모델을 훨씬 뛰어넘는 검색 시스템 BM25. 단일 언어 검색 작업이 대규모로부터 이익을 얻은 반면 MS MARCO와 같은 훈련 컬렉션과 신경 아키텍처의 발전, 교차 언어 검색 작업은 이러한 발전보다 뒤처져 왔다. 이것. 논문은 ColBERT의 일반화인 ColBERT-X를 소개한다. XLM-RoBERTa(XLM-R)를 사용하는 다중 표현 밀도 검색 모델 CLIR(Cross Language Information Research)을 지원하는 인코더. 콜버트-X 캔 두 가지 방법으로 훈련되다 제로샷 교육에서 시스템은 영어 MS MARCO 모음, 교차 언어용 XLM-R 인코더에 의존 매핑. 번역 열차에서 시스템은 MS MARCO 영어에 대해 교육됩니다. 관련 MS MARCO 구문의 기계 번역과 결합된 쿼리. 여러 언어로 된 임시 문서 순위 지정 작업에 대한 결과는 다음을 입증한다. 이러한 훈련된 밀도의 실질적이고 통계적으로 유의한 개선 기존 어휘 CLIR 기준선을 통해 모델을 검색합니다.
Both the validity of the cultural tightness index and the association with creativity and order are spurious -- a comment on Jackson et al
It was recently suggested in a study published in Nature Human Behaviour that the historical loosening of American culture was associated with a trade-off between higher creativity and lower order. To this end, Jackson et al. generate a linguistic index of cultural tightness based on the Google Books Ngram corpus and use this index to show that American norms loosened between 1800 and 2000. While we remain agnostic toward a potential loosening of American culture and a statistical association with creativity/order, we show here that the methods used by Jackson et al. are neither suitable for testing the validity of the index nor for establishing possible relationships with creativity/order.
최근 Nature Human Behavior에 발표된 연구에서 다음과 같이 제안되었다. 미국 문화의 역사적 이완은 절충과 관련이 있었다. 높은 창의력과 낮은 질서 사이에서 말이죠. 이를 위해 잭슨 등이 제작한다. 구글 북스 엔그램 코퍼스에 기반한 문화적 긴밀성의 언어 지수 1800년과 2000년 사이에 미국의 규범이 느슨해졌다는 것을 보여주기 위해 이 지수를 사용한다. 우리는 미국 문화의 잠재적인 이완과 미국 문화에 대해 불가지론적인 입장을 견지하고 있다. 창의성/질서와의 통계적 연관성, 우리는 여기서 그 방법들을 보여준다. 잭슨 등에 의해 사용된 것은 그 타당성을 시험하는데 적합하지 않다. 창의성/질서와의 관계를 설정하기 위한 지표도 아니다.
The ABBE Corpus: Animate Beings Being Emotional
Emotion detection is an established NLP task of demonstrated utility for text understanding. However, basic emotion detection leaves out key information, namely, who is experiencing the emotion in question. For example, it may be the author, the narrator, or a character; or the emotion may correspond to something the audience is supposed to feel, or even be unattributable to a specific being, e.g., when emotions are being discussed per se. We provide the ABBE corpus -- Animate Beings Being Emotional -- a new double-annotated corpus of texts that captures this key information for one class of emotion experiencer, namely, animate beings in the world described by the text. Such a corpus is useful for developing systems that seek to model or understand this specific type of expressed emotion. Our corpus contains 30 chapters, comprising 134,513 words, drawn from the Corpus of English Novels, and contains 2,010 unique emotion expressions attributable to 2,227 animate beings. The emotion expressions are categorized according to Plutchik's 8-category emotion model, and the overall inter-annotator agreement for the annotations was 0.83 Cohen's Kappa, indicating excellent agreement. We describe in detail our annotation scheme and procedure, and also release the corpus for use by other researchers.
감정 감지는 텍스트에 대해 입증된 효용의 확립된 NLP 작업이다. 이해 하지만 기본적인 감정 감지는 중요한 정보를 생략하고 즉, 누가 문제의 감정을 경험하고 있는가. 예를 들어 다음과 같은 식이 될 수 있습니다. 작가, 서술자, 또는 등장인물; 또는 감정은 일치할 수 있다 청중이 느끼도록 되어 있거나 심지어 매력적이지 않은 것 예를 들어, 감정이 그 자체로 논의될 때, 특정한 존재. 우리는 다음을 제공한다 ABBE 말뭉치 - 감정이입된 생물체 - 새로운 이중 주석 말뭉치 한 종류의 감정에 대한 이 핵심 정보를 포착하는 텍스트들 경험자, 즉 텍스트에 의해 묘사된 세계의 생물체들. 그런. 말뭉치는 이것을 모델링하거나 이해하려는 시스템을 개발하는데 유용하다. 표현된 감정의 특정 유형. 우리 말뭉치는 30개의 장을 포함하고 있다. 134,513개의 단어가 영어 소설 코퍼스에서 발췌되었고 2,010개를 포함하고 있다. 2,227개의 생물체에 기인하는 독특한 감정 표현들 감정 표현은 플루틱의 8가지 범주 감정 모델에 따라 분류된다. 그리고 주석에 대한 전체 주석자간 합치도는 0.83 Cohen의 것이었다. 카파: 합치도가 매우 높다는 것을 나타냅니다. 우리는 우리의 주석을 자세히 설명한다. 체계와 절차, 그리고 또한 다른 연구자들이 사용할 수 있도록 말뭉치를 공개한다.
'paper-of-the-day' 카테고리의 다른 글
[2022-02-04] 오늘의 자연어처리 (0) | 2022.02.04 |
---|---|
[2022-02-03] 오늘의 자연어처리 (0) | 2022.02.03 |
[2022-01-27] 오늘의 자연어처리 (0) | 2022.01.27 |
[2022-01-26] 오늘의 자연어처리 (0) | 2022.01.26 |
[2022-01-25] 오늘의 자연어처리 (0) | 2022.01.25 |